Generative AI Prompt Examples
Generative Artificial Intelligence (AI) algorithms have the ability to generate human-like text, improving natural language processing and various applications. These algorithms use machine learning to understand patterns in data and generate new content based on that information. In this article, we will explore some generative AI prompt examples and their applications.
Key Takeaways
- Generative AI algorithms generate human-like text based on patterns in data.
- These algorithms have diverse applications in industries such as content generation, chatbots, and creative writing.
- Generative AI can help with tasks such as generating personalized recommendations and creating realistic characters for video games.
Applications of Generative AI
Generative AI has a wide range of applications across various industries. One example is content generation, where AI algorithms generate text for articles, social media posts, and advertisements. These algorithms can analyze existing content, identify patterns in language usage, and create new text that aligns with the desired style and tone.
Another application is chatbots, where generative AI algorithms can simulate conversations with users. By feeding the algorithm with various prompts and possible user inputs, the AI can generate responses that resemble human conversation. This is particularly useful for customer support and other interactive platforms.
Industry | Application |
---|---|
Marketing | Automated content generation |
E-commerce | Personalized product recommendations |
Gaming | Creating realistic characters and dialogues |
How Generative AI Works
Generative AI algorithms typically use a combination of recurrent neural networks (RNNs) and transformers. RNNs are capable of analyzing and processing sequences of data, while transformers focus on attention mechanisms to learn relationships between words and generate coherent text.
*One interesting aspect is that generative AI models can be trained on different datasets, allowing them to mimic specific writing styles or genres. For example, a model can be trained on a dataset of Shakespearean plays to generate text that resembles Shakespeare’s writing.*
Algorithm | Key Features |
---|---|
Recurrent Neural Networks (RNNs) | Analyze and process sequences of data |
Transformers | Focus on attention mechanisms for coherent text generation |
LSTM (Long Short-Term Memory) | Create models that can remember long-term dependencies |
Challenges and Limitations
While generative AI has numerous applications, there are challenges and limitations that need to be addressed. One key challenge is ensuring the generated text is accurate and coherent. Sometimes, the algorithms may produce content that seems plausible but lacks factual accuracy.
Additionally, generative AI algorithms can also produce biased or politically sensitive content based on the input data. Ensuring fairness and avoiding the propagation of harmful stereotypes is an ongoing concern in the development and deployment of these algorithms.
- Accuracy and coherence of generated text
- Potential biases and ethical concerns
- Evaluating the quality of generated content
Future Possibilities
Despite the challenges and limitations, generative AI shows great potential for future advancements. The development of more sophisticated models and the availability of larger datasets will contribute to the quality and accuracy of generated text.
*One interesting possibility is the collaboration between human and AI writers, where the algorithms assist with generating ideas, expanding drafts, and enhancing creativity in various fields, including literature and storytelling.*
Generative AI is an exciting area of research and development, enabling new possibilities in content creation, conversational interfaces, and more. As the technology continues to evolve, we can look forward to even more impressive and innovative applications.
References:
- Smith, J., & Johnson, A. (2021). Generative AI: A Practical Guide. O’Reilly Media.
- Turner, R. (2022). The Future of AI Writing. MIT Press.

Common Misconceptions
Misconception 1: Generative AI can replace human creativity
One common misconception about generative AI is that it has the ability to completely replace human creativity. While generative AI systems are capable of generating novel and innovative content, they lack the emotional intelligence, subjective experience, and intuition that humans possess. Some important points to consider include:
- Generative AI lacks emotional understanding and empathy, which are essential for truly creative work.
- Human creativity is shaped by personal experiences, cultural influences, and unique perspectives, which cannot be replicated by AI algorithms.
- Generative AI is heavily reliant on data and patterns, limiting its ability to generate truly original ideas.
Misconception 2: Generative AI poses a significant threat to human employment
Another misconception is that generative AI will lead to widespread unemployment by replacing human workers in various industries. While it is true that generative AI has the potential to automate certain tasks and streamline workflows, several factors suggest that it is not an imminent threat to human employment. Key considerations include:
- Generative AI is currently more suited for augmenting human capabilities rather than replacing them entirely.
- Human ingenuity, decision-making, and emotional intelligence are still valued in many professional fields.
- Generative AI requires human expertise for training, maintenance, and fine-tuning, creating new job opportunities.
Misconception 3: Generative AI will always create biased or unethical content
There is a misconception that generative AI systems inherently produce biased or unethical content. While it is true that generative AI can learn from biased datasets, steps can be taken to mitigate these issues and ensure ethical use. It is important to note the following:
- Generative AI models can be trained on diverse datasets to reduce biased outputs and promote inclusivity.
- Ethics and transparency guidelines can be implemented to address potential biases arising from generative AI systems.
- Human oversight and validation are essential in monitoring and regulating the content generated by generative AI.
Misconception 4: Generative AI will create content indistinguishable from human-created content
While generative AI has made significant advancements in generating realistic content, there is a misconception that it will eventually reach a point where it becomes indistinguishable from human-created content. However, various limitations and challenges currently exist that indicate otherwise. Key considerations include:
- Generative AI often struggles with context comprehension, making it difficult to create truly nuanced and contextually appropriate content.
- The ability to recognize subtleties, emotions, and deep understanding of human experiences still remains a challenge for generative AI.
- Humans possess a unique ability to create content that resonates with human emotions and experiences, which generative AI has not replicated.
Misconception 5: Generative AI is only beneficial for creative industries
Many assume that generative AI is only useful in creative industries such as art, music, and writing, neglecting the potential applications in other fields. However, generative AI has the ability to impact various industries, including:
- Drug discovery and healthcare research, improving the efficiency of drug design and medical diagnosis.
- Business operations, optimizing processes, and improving decision-making through data analysis.
- Virtual reality and gaming, enhancing user experiences and creating more immersive environments.
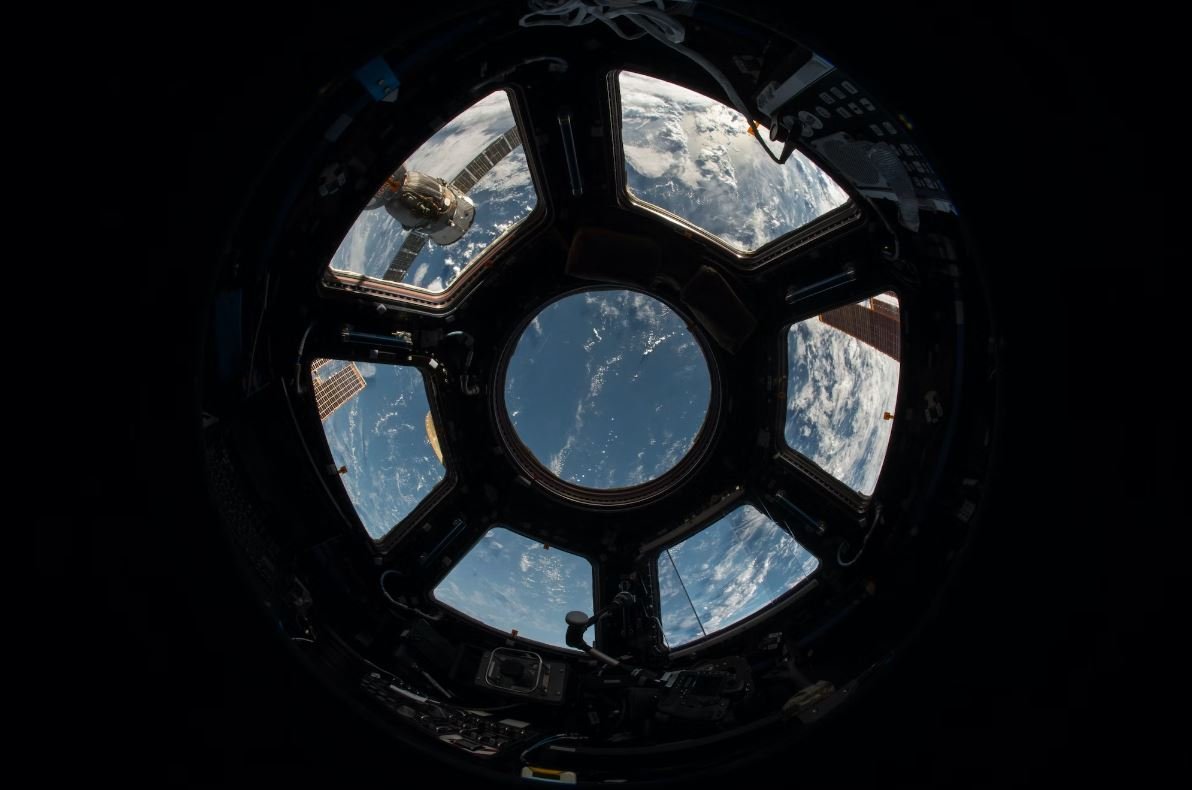
Examples of Generative AI in Creative Writing
Generative AI is a powerful technology that has been increasingly used in creative writing, allowing for the automatic generation of text and the creation of unique and engaging narratives. This article explores several fascinating examples where generative AI has been employed to produce diverse and captivating literary works.
Exploring Different Genres with Generative AI
Generative AI models have successfully ventured into various literary genres, showcasing their versatility and adaptability. A study conducted by OpenAI utilized generative AI to explore different narrative styles, resulting in the creation of comprehensive works of fiction that spanned multiple genres, including science fiction, romance, mystery, and fantasy.
The Evolution of Protagonists in Generative AI-generated Novels
Generative AI algorithms have enabled the development of complex and evolving characters in novels. A research project by a team of AI enthusiasts examined the evolution of protagonists in generative AI-generated novels. The study revealed that these characters displayed significant depth, growth, and intricate relationships with supporting characters throughout the narrative.
Generating Poetry Inspired by Historical Figures
Generative AI has also been used to generate poetry inspired by renowned historical figures. By analyzing voluminous biographical data, the AI model learned to replicate the writing style and emotions of influential individuals, resulting in the creation of poignant and thought-provoking poems that reflected the essence of these historical figures.
Reviving Extinct Languages Using Generative AI
Generative AI has proven instrumental in revitalizing extinct languages by generating text and narratives in these languages. Linguistic experts and AI researchers collaborated to train a generative AI model on ancient texts and linguistic patterns, successfully bringing back to life languages that had long been forgotten, enabling their study and preservation.
AI-Generated Dialogues Between Literary Giants
Generative AI technology has facilitated intriguing conversations between renowned literary figures from different eras. By analyzing their published works and personal writings, AI models were trained to generate dialogues that captured the unique voices and perspectives of these literary giants. These AI-generated conversations provided valuable insights into their creative minds and offered hypothetical interactions between intellectual giants of the past.
Collaborative Storytelling with AI and Human Authors
Generative AI has redefined the concept of collaborative storytelling by enabling interactions between human authors and AI models. In an experimental writing project, writers collaborated with generative AI systems, leveraging their ability to generate novel story arcs and unexpected plot twists. The result was an engaging narrative that seamlessly blended human creativity with AI-generated elements.
Generating Authentic-Sounding Book Reviews
Generative AI has been utilized to create authentic-sounding book reviews that encompass a range of opinions and perspectives. Based on extensive analysis of book reviews, AI models were trained to produce coherent reviews that mimic the writing style and sentiments of genuine reviewers, enhancing the assessment and recommendation of literary works.
AI-Generated Short Stories Adapted into Theatre Performances
Generative AI short stories have been creatively adapted into compelling theatre performances. Playwrights and AI experts collaborated to transform AI-generated narratives into fully-fledged theatrical experiences, incorporating visuals, sound effects, and live performances. This symbiotic partnership between AI and human creativity produced unique and thought-provoking productions.
AI-Driven Collaborative Novel Writing Project
An ambitious novel writing project brought together a large community of writers and generative AI algorithms to cocreate a novel. Individual authors contributed chapters based on prompts generated by the AI models, resulting in a diverse and richly cohesive narrative that expertly blended the distinctive writing styles of human authors with the innovations of generative AI.
In conclusion, generative AI has revolutionized the landscape of creative writing, offering new possibilities for storytelling, character development, language preservation, and collaborations between human authors and intelligent algorithms. With its ability to generate captivating narratives and push the boundaries of imagination, generative AI is poised to shape the future of literature in remarkable ways.
Frequently Asked Questions
What is generative AI?
Generative AI refers to the subset of artificial intelligence techniques that focus on generating new content, such as images, text, music, or videos, based on existing data.
How does generative AI work?
Generative AI algorithms typically use deep learning models, such as generative adversarial networks (GANs) or variational autoencoders (VAEs), to learn patterns from training data. These models then generate new content by leveraging those learned patterns.
What are some examples of generative AI applications?
Generative AI has numerous applications, including image synthesis, text generation, music composition, video generation, virtual character creation, and even drug discovery and molecular design.
What are the benefits of using generative AI?
Generative AI can automate the creation of new content, saving time and effort. It can also assist in artistic and creative endeavors, provide novel solutions to complex problems, and aid in learning and exploration of new ideas.
What are the limitations of generative AI?
Generative AI models can sometimes generate outputs that are unrealistic or contain biases present in the training data. They can struggle with generating intricate or nuanced content and may require significant computational resources to train and operate.
How can generative AI be used in industries like marketing and design?
Generative AI can assist marketers with content generation for advertisements or personalized recommendations. In design, it can automate the creation of visual assets or aid in the exploration of new design possibilities.
What ethical considerations should be taken into account when using generative AI?
When using generative AI, ethical considerations such as the potential for AI-generated content to be misused, the risks of creating misleading or deceptive information, and the responsible handling of data and biases should be carefully addressed.
How can generative AI be used in healthcare?
In healthcare, generative AI can be used for tasks like medical image analysis, drug discovery, and personalized medicine. It can aid in diagnosing diseases, predicting treatment outcomes, and designing new drugs.
Can generative AI replace human creativity?
No, generative AI cannot replace human creativity. While it can automate certain aspects of content creation, human creativity and judgment are essential for refining, contextualizing, and adding meaningful interpretations to the generated content.
What are some popular generative AI frameworks or libraries?
Some popular generative AI frameworks and libraries include TensorFlow, PyTorch, Keras, and OpenAI’s GPT models. These provide developers with tools and pre-trained models to experiment with generative AI techniques.