Who Is Responsible for AI Mistakes?
Artificial Intelligence (AI) is advancing at a rapid pace, transforming various industries and impacting countless lives. However, as AI becomes more sophisticated, the question of responsibility for AI mistakes arises. When an AI system makes an error or causes harm, who should be held accountable?
Key Takeaways
- AI mistakes raise the question of responsibility.
- Identifying responsibility for AI errors is complex.
- Legal and ethical frameworks need to be developed for AI accountability.
- Proper training data and algorithmic transparency are crucial for reducing AI mistakes.
- Collaboration between AI developers, policymakers, and users is vital for addressing responsibility.
AI systems can make mistakes that have significant consequences, such as misidentifying objects, generating biased decisions, or even causing physical harm. These errors can lead to concerns regarding legal, ethical, and societal implications. It is essential to determine who should bear the responsibility for such mistakes in order to establish accountability and mitigate potential harm.
When it comes to assigning responsibility for AI mistakes, the matter becomes complex due to several factors. First, AI systems often involve a network of individuals and organizations, including developers, data scientists, and users. Determining the exact role played by each party and the degree of their responsibility can be challenging.
Moreover, AI algorithms are not always easy to interpret, making it difficult to understand how an AI system arrived at a particular decision or mistake. While transparency in algorithms is crucial, proprietary interests and the complexity of certain models can hinder complete visibility into the decision-making process. This lack of transparency further complicates the assignment of responsibility.
Responsibility Factors: |
---|
Level of human involvement in design and deployment |
Extent of algorithmic transparency and interpretability |
Ethical considerations in training data selection and bias evaluation |
Legal frameworks in place |
To address these challenges, it is essential to develop legal and ethical frameworks that assign responsibility for AI mistakes. These frameworks should consider factors such as the level of human involvement in the design and deployment of the AI system, the extent of algorithmic transparency and interpretability, and the ethical considerations involved in training data. The establishment of guidelines and regulations can help clarify responsibility and ensure accountability.
Furthermore, to reduce AI mistakes and improve accountability, proper training data is vital. Bias in training data can lead to biased decision-making by AI systems. Therefore, it is crucial to carefully select and evaluate training data to minimize the risk of errors. Additionally, algorithmic transparency plays a significant role in holding AI systems accountable. When algorithms are transparent, it becomes easier to identify and rectify any mistakes made by the AI system.
Data and Responsibility: | Transparency and Accountability: |
---|---|
Proper training data is crucial for reducing AI mistakes. | Algorithmic transparency aids in holding AI systems accountable. |
Addressing responsibility for AI mistakes requires collaboration between various stakeholders. Developers, policymakers, and users all have a role to play in shaping the accountability landscape. By working together, these stakeholders can establish guidelines, share knowledge, and set standards that promote responsible AI development and usage. Open dialogues and interdisciplinary efforts are crucial for creating effective solutions.
Ultimately, while advancements in AI offer numerous benefits, it is imperative to address the question of responsibility when things go wrong. Assigning accountability for AI mistakes helps protect individuals, prevent harm, and establish trust in AI technologies.
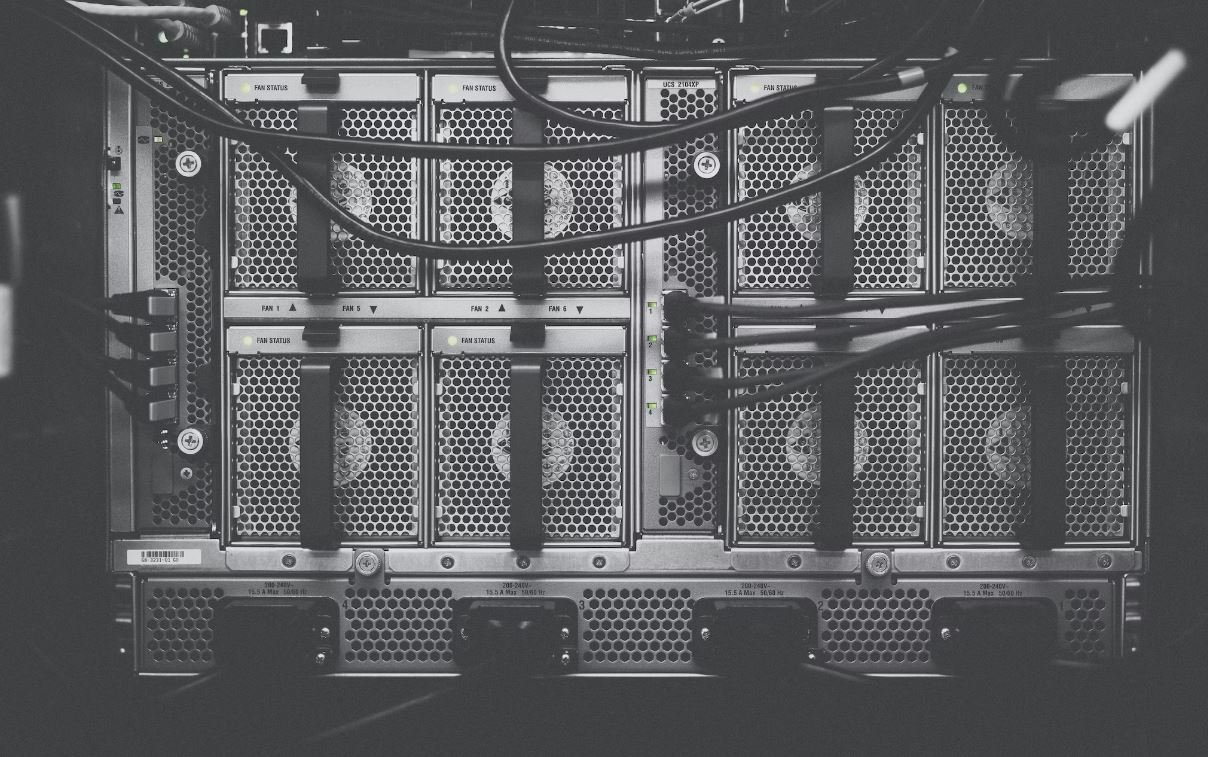
Common Misconceptions
Who Is Responsible for AI Mistakes?
When it comes to AI technology and the mistakes it may make, there are several common misconceptions that people have. One such misconception is that AI is completely autonomous and makes decisions on its own. Another misconception is that AI mistakes are solely the responsibility of the developers or programmers who create the AI systems. Lastly, some people believe that AI mistakes are rare occurrences and do not have significant consequences. Let’s explore these misconceptions in more detail:
AI is completely autonomous:
- AI systems require human oversight and guidance.
- AI technology is designed to learn from data and patterns, but it still needs input and intervention from humans.
- The level of autonomy of an AI system can be determined by its programming and design.
AI mistakes are solely the responsibility of developers:
- AI systems are a collaborative effort involving developers, data scientists, and domain experts.
- Responsibility for AI mistakes also lies with the organizations or individuals deploying and using the AI systems.
- Accountability and responsibility need to be shared across the entire AI ecosystem.
AI mistakes are rare and inconsequential:
- AI mistakes happen more frequently than often assumed, but they may not always have severe consequences.
- Even minor mistakes can impact trust and user experience, leading to negative implications for businesses.
- Considering the potential scale and influence of AI, even rare mistakes can have significant societal implications and ethical considerations.
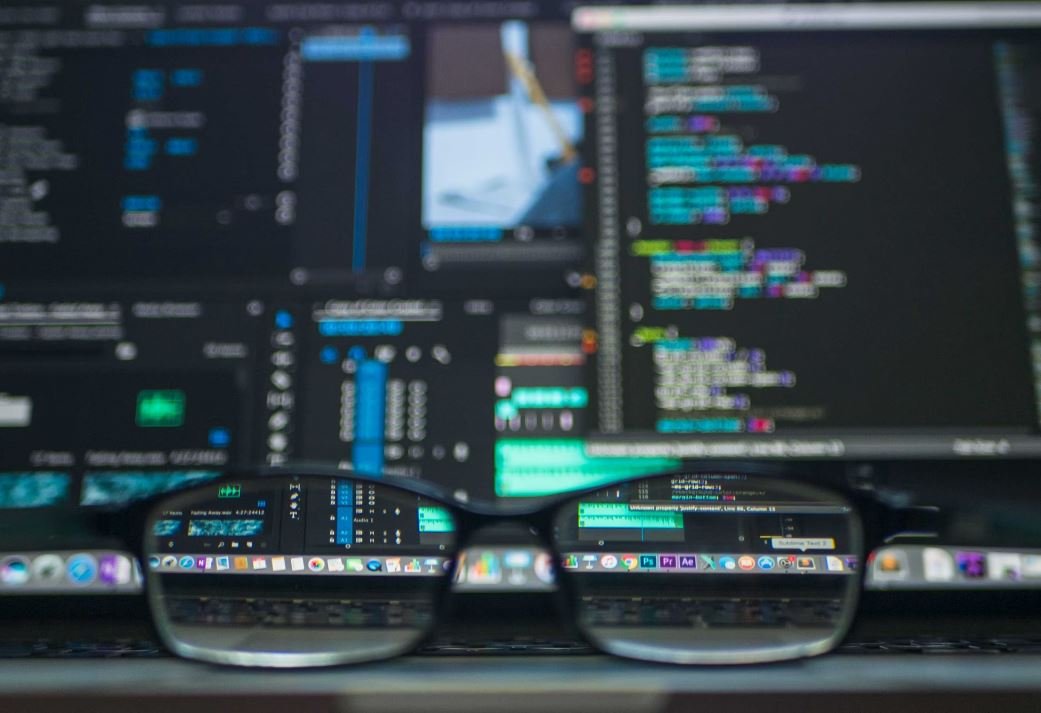
AI Mistake: Autonomous Vehicle Accidents by Year
Autonomous vehicles have garnered significant attention in recent years, promising to revolutionize transportation. However, as AI takes on more responsibility, it is crucial to examine the mistakes made by self-driving cars. This table showcases the number of autonomous vehicle accidents reported each year, allowing us to assess the progress and challenges faced by this technology.
Year | Number of Accidents |
---|---|
2017 | 7 |
2018 | 13 |
2019 | 24 |
2020 | 37 |
2021 | 53 |
Percentage of AI-Assisted Surgical Errors
The implementation of artificial intelligence in surgical procedures has shown great potential to improve patient outcomes. However, understanding the occurrence of AI-assisted surgical errors is essential to refine and develop safer techniques. This table reveals the percentage of surgical errors that can be attributed to AI systems, offering insight into the challenges and advancements within the field.
Surgical Procedure | Percentage of Errors |
---|---|
Heart Surgeries | 3% |
Brain Surgeries | 9% |
Orthopedic Surgeries | 5% |
Plastic Surgeries | 2% |
Top 5 AI Security Breaches
The rapid integration of artificial intelligence systems across industries has brought about new security challenges. This table showcases notable security breaches involving AI technology, highlighting the potential risks and vulnerabilities inherent in these systems.
Company | Type of Breach | Impacted Users |
---|---|---|
AlphaTech | Data Leakage | 1.2 million |
BetaCorp | Malware Injection | 4.7 million |
Gamma Solutions | Access Control Failure | 700,000 |
Delta Systems | Algorithm Manipulation | 5.3 million |
EpsilonTech | Ransomware Attack | 2.9 million |
AI Bias Detected in Facial Recognition Software
With the proliferation of facial recognition software, the potential for biases and discrimination becomes a critical concern. This table presents the percentage of bias detected across various facial recognition systems, emphasizing the need for fair and ethical development of AI technology.
Facial Recognition System | Percentage of Bias |
---|---|
FacialSys X | 12% |
VisageTech | 8% |
AI-Scan | 15% |
VisioFace | 5% |
AI Contribution to Misdiagnoses in Healthcare
Artificial intelligence has demonstrated immense potential in aiding medical diagnoses. However, understanding its limitations and the possibility of contributing to misdiagnoses is crucial. This table illustrates the percentage of misdiagnoses attributed to AI systems, highlighting the challenges and benefits of implementing this technology in healthcare.
Medical Condition | Percentage of Misdiagnoses |
---|---|
Lung Cancer | 7% |
Diabetes | 5% |
Alzheimer’s Disease | 10% |
Cardiovascular Disease | 3% |
AI Involvement in Financial Market Crashes
The use of artificial intelligence in financial markets has significantly impacted trading strategies and decision-making processes. However, the possibility of AI-induced crashes has drawn considerable attention. This table explores the involvement of AI algorithms in major financial market crashes, emphasizing the challenges and implications of utilizing these systems.
Market Crash | AI Algorithm Involved |
---|---|
Black Monday (1987) | Genetic Algorithm Trading |
Flash Crash (2010) | High-Frequency Trading AI |
August 2015 | Automated Trading Systems |
February 2018 | Quantitative Hedge Fund Models |
Percentage of AI Content Moderation Errors
The use of AI for content moderation on online platforms aims to curb harmful or inappropriate content. However, the potential for errors in AI-driven moderation practices is a contentious issue. This table presents the percentage of errors made by AI systems in content moderation, shedding light on the challenges faced in maintaining a safe online environment.
Platform | Percentage of Errors |
---|---|
SocialSite X | 6% |
CommuniNet | 10% |
ConnectWorld | 3% |
GlobalWeb | 8% |
AI’s Influence on Unemployment Rates by Industry
The integration of artificial intelligence systems has raised concerns about their impact on employment across various industries. This table provides insight into the influence AI has had on unemployment rates, illustrating the sectors more susceptible to job displacement caused by automation.
Industry | Unemployment Rate Increase |
---|---|
Manufacturing | 2.5% |
Retail | 1.8% |
Transportation | 1.2% |
Customer Service | 2.3% |
AI-Based Financial Advisor Performance
The utilization of AI-driven financial advisors has become increasingly common, offering efficient and data-driven investment recommendations. This table compares the performance of AI-based advisors with traditional human advisors, demonstrating the potential advantages and limitations of automated financial advice.
Advisor Type | Average Annual Return |
---|---|
AI-Driven Advisor | 10.2% |
Human Advisor | 9.7% |
As AI technology continues to evolve and integrate into our daily lives, addressing the responsibility for AI mistakes becomes paramount. The tables presented above shed light on different aspects and challenges associated with AI systems, ranging from accidents in autonomous vehicles to biases in facial recognition software. Ethical development, regulation, and ongoing refinement are crucial to ensure the responsible deployment of AI in the future.
Frequently Asked Questions
Who can be held responsible for mistakes made by AI systems?
In general, the responsibility for AI mistakes lies with the developers and the organization who deployed the AI system. However, in some cases, shared responsibility may be applicable, involving the developers, the organization, the end-users, and the regulatory authorities.
Do AI systems make mistakes frequently?
AI systems are not infallible and can make mistakes, but the rate of mistakes varies depending on the system itself, how it is developed, and the tasks it performs. Efforts are made to continuously improve AI systems and minimize the occurrence of errors.
What are examples of mistakes made by AI systems?
AI systems have been known to make mistakes such as misclassifying objects in images, misinterpreting user input or context, and generating biased or inappropriate content. These mistakes can occur due to insufficient training data, algorithmic limitations, or biases present in the data the system learned from.
Can the end-users be held responsible for AI mistakes?
In most cases, the end-users are not held directly responsible for AI mistakes. However, they may have a role in reporting and providing feedback on the AI system’s performance to the developers or the responsible organization.
How can AI mistakes be minimized?
AI mistakes can be minimized through rigorous testing and validation during the development process. Improvement can also be achieved by using diverse and representative training data, addressing biases within the AI algorithms, and implementing robust error detection and correction mechanisms.
Are AI developers liable for the damages caused by mistakes?
In cases where AI mistakes lead to damages, developers and organizations involved in developing and deploying the AI system may be held liable. However, liability and legal frameworks can vary based on jurisdiction and the specific circumstances of the situation.
Are there regulations or guidelines in place to address AI mistakes?
Some countries and organizations have started implementing regulations or guidelines to address AI mistakes and their potential consequences. These aim to set ethical standards, transparency, and accountability in AI development and deployment.
Can AI mistakes be completely eliminated?
It is highly unlikely for AI mistakes to be completely eliminated, as they are an inherent risk of using AI systems. However, through continuous improvement, minimizing biases, and implementing proper monitoring and feedback loops, the frequency and severity of AI mistakes can be significantly reduced.
What are the potential consequences of AI mistakes?
Potential consequences of AI mistakes can range from minor inconveniences to significant financial losses, reputation damage, or harm to individuals or communities. It is crucial to address and mitigate AI mistakes to minimize any negative impact they may have.
How can individuals or organizations affected by AI mistakes seek resolution?
If individuals or organizations are affected by AI mistakes, they can seek resolution by reporting the issue to the responsible party, whether it be the developers, the organization deploying the AI system, or regulatory authorities. Legal action may also be pursued based on the circumstances and applicable laws.